mirror of
https://github.com/ggerganov/llama.cpp.git
synced 2025-01-15 23:00:46 +01:00
514 lines
23 KiB
Markdown
514 lines
23 KiB
Markdown
![]() |
|
||
|

|
||
|

|
||
|

|
||
|

|
||
|

|
||
|
[](https://bestpractices.coreinfrastructure.org/projects/4834)
|
||
|
|
||
|
<table>
|
||
|
<tr>
|
||
|
|
||
|
<td width="20%">
|
||
|
<img src="https://raw.githubusercontent.com/KomputeProject/kompute/master/docs/images/kompute.jpg">
|
||
|
</td>
|
||
|
|
||
|
<td>
|
||
|
|
||
|
<h1>Kompute</h1>
|
||
|
<h3>The general purpose GPU compute framework for cross vendor graphics cards (AMD, Qualcomm, NVIDIA & friends)</h3>
|
||
|
|
||
|
</td>
|
||
|
|
||
|
</tr>
|
||
|
</table>
|
||
|
|
||
|
<h4>Blazing fast, mobile-enabled, asynchronous, and optimized for advanced GPU acceleration usecases.</h4>
|
||
|
|
||
|
💬 [Join the Discord & Community Calls](https://kompute.cc/overview/community.html) 🔋 [Documentation](https://kompute.cc) 💻 [Blog Post](https://medium.com/@AxSaucedo/machine-learning-and-data-processing-in-the-gpu-with-vulkan-kompute-c9350e5e5d3a) ⌨ [Examples](#more-examples) 💾
|
||
|
|
||
|
<hr>
|
||
|
|
||
|
##### Kompute is backed by the Linux Foundation as a <a href="https://lfaidata.foundation/blog/2021/08/26/kompute-joins-lf-ai-data-as-new-sandbox-project/">hosted project</a> by the LF AI & Data Foundation.
|
||
|
|
||
|
<table>
|
||
|
<tr>
|
||
|
<td>
|
||
|
<a href="https://www.linuxfoundation.org/projects/">
|
||
|
<img src="https://upload.wikimedia.org/wikipedia/commons/b/b5/Linux_Foundation_logo.png">
|
||
|
</a>
|
||
|
</td>
|
||
|
<td>
|
||
|
<a href="https://lfaidata.foundation/projects/">
|
||
|
<img src="https://raw.githubusercontent.com/lfai/artwork/main/lfaidata-assets/lfaidata/horizontal/color/lfaidata-horizontal-color.png">
|
||
|
</a>
|
||
|
</td>
|
||
|
</tr>
|
||
|
</table>
|
||
|
|
||
|
|
||
|
## Principles & Features
|
||
|
|
||
|
* [Flexible Python module](#your-first-kompute-python) with [C++ SDK](#your-first-kompute-c) for optimizations
|
||
|
* [Asynchronous & parallel processing](#asynchronous-and-parallel-operations) support through GPU family queues
|
||
|
* [Mobile enabled](#mobile-enabled) with examples via Android NDK across several architectures
|
||
|
* BYOV: [Bring-your-own-Vulkan design](#motivations) to play nice with existing Vulkan applications
|
||
|
* Explicit relationships for GPU and host [memory ownership and memory management](https://kompute.cc/overview/memory-management.html)
|
||
|
* Robust codebase with [90% unit test code coverage](https://kompute.cc/codecov/)
|
||
|
* Advanced use-cases on [machine learning 🤖](https://towardsdatascience.com/machine-learning-and-data-processing-in-the-gpu-with-vulkan-kompute-c9350e5e5d3a), [mobile development 📱](https://towardsdatascience.com/gpu-accelerated-machine-learning-in-your-mobile-applications-using-the-android-ndk-vulkan-kompute-1e9da37b7617) and [game development 🎮](https://towardsdatascience.com/supercharging-game-development-with-gpu-accelerated-ml-using-vulkan-kompute-the-godot-game-engine-4e75a84ea9f0).
|
||
|
* Active community with [monthly calls, discord chat and more](https://kompute.cc/overview/community.html)
|
||
|
|
||
|
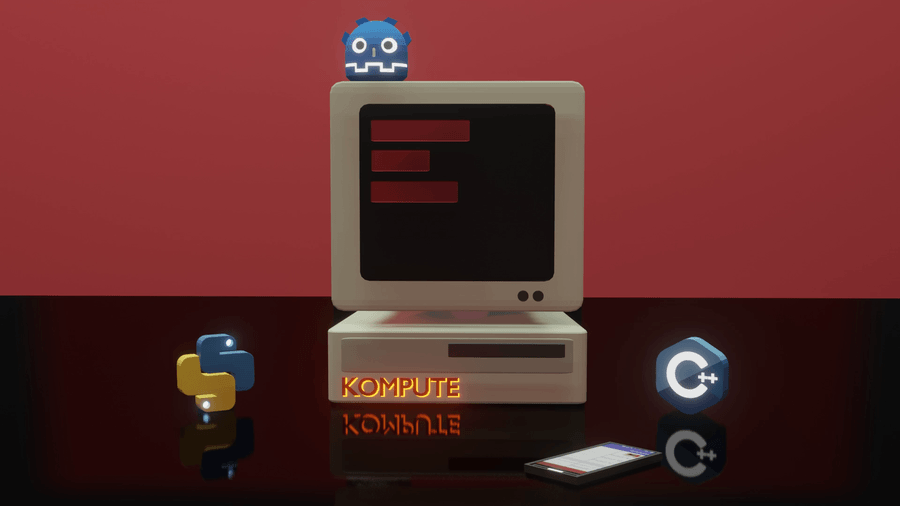
|
||
|
|
||
|
## Getting Started
|
||
|
|
||
|
Below you can find a GPU multiplication example using the C++ and Python Kompute interfaces.
|
||
|
|
||
|
You can [join the Discord](https://discord.gg/MaH5Jv5zwv) for questions / discussion, open a [github issue](https://github.com/KomputeProject/kompute/issues/new), or read [the documentation](https://kompute.cc/).
|
||
|
|
||
|
### Your First Kompute (C++)
|
||
|
|
||
|
The C++ interface provides low level access to the native components of Kompute, enabling for [advanced optimizations](https://kompute.cc/overview/async-parallel.html) as well as [extension of components](https://kompute.cc/overview/reference.html).
|
||
|
|
||
|
```c++
|
||
|
|
||
|
void kompute(const std::string& shader) {
|
||
|
|
||
|
// 1. Create Kompute Manager with default settings (device 0, first queue and no extensions)
|
||
|
kp::Manager mgr;
|
||
|
|
||
|
// 2. Create and initialise Kompute Tensors through manager
|
||
|
|
||
|
// Default tensor constructor simplifies creation of float values
|
||
|
auto tensorInA = mgr.tensor({ 2., 2., 2. });
|
||
|
auto tensorInB = mgr.tensor({ 1., 2., 3. });
|
||
|
// Explicit type constructor supports uint32, int32, double, float and bool
|
||
|
auto tensorOutA = mgr.tensorT<uint32_t>({ 0, 0, 0 });
|
||
|
auto tensorOutB = mgr.tensorT<uint32_t>({ 0, 0, 0 });
|
||
|
|
||
|
std::vector<std::shared_ptr<kp::Tensor>> params = {tensorInA, tensorInB, tensorOutA, tensorOutB};
|
||
|
|
||
|
// 3. Create algorithm based on shader (supports buffers & push/spec constants)
|
||
|
kp::Workgroup workgroup({3, 1, 1});
|
||
|
std::vector<float> specConsts({ 2 });
|
||
|
std::vector<float> pushConstsA({ 2.0 });
|
||
|
std::vector<float> pushConstsB({ 3.0 });
|
||
|
|
||
|
auto algorithm = mgr.algorithm(params,
|
||
|
// See documentation shader section for compileSource
|
||
|
compileSource(shader),
|
||
|
workgroup,
|
||
|
specConsts,
|
||
|
pushConstsA);
|
||
|
|
||
|
// 4. Run operation synchronously using sequence
|
||
|
mgr.sequence()
|
||
|
->record<kp::OpTensorSyncDevice>(params)
|
||
|
->record<kp::OpAlgoDispatch>(algorithm) // Binds default push consts
|
||
|
->eval() // Evaluates the two recorded operations
|
||
|
->record<kp::OpAlgoDispatch>(algorithm, pushConstsB) // Overrides push consts
|
||
|
->eval(); // Evaluates only last recorded operation
|
||
|
|
||
|
// 5. Sync results from the GPU asynchronously
|
||
|
auto sq = mgr.sequence();
|
||
|
sq->evalAsync<kp::OpTensorSyncLocal>(params);
|
||
|
|
||
|
// ... Do other work asynchronously whilst GPU finishes
|
||
|
|
||
|
sq->evalAwait();
|
||
|
|
||
|
// Prints the first output which is: { 4, 8, 12 }
|
||
|
for (const float& elem : tensorOutA->vector()) std::cout << elem << " ";
|
||
|
// Prints the second output which is: { 10, 10, 10 }
|
||
|
for (const float& elem : tensorOutB->vector()) std::cout << elem << " ";
|
||
|
|
||
|
} // Manages / releases all CPU and GPU memory resources
|
||
|
|
||
|
int main() {
|
||
|
|
||
|
// Define a raw string shader (or use the Kompute tools to compile to SPIRV / C++ header
|
||
|
// files). This shader shows some of the main components including constants, buffers, etc
|
||
|
std::string shader = (R"(
|
||
|
#version 450
|
||
|
|
||
|
layout (local_size_x = 1) in;
|
||
|
|
||
|
// The input tensors bind index is relative to index in parameter passed
|
||
|
layout(set = 0, binding = 0) buffer buf_in_a { float in_a[]; };
|
||
|
layout(set = 0, binding = 1) buffer buf_in_b { float in_b[]; };
|
||
|
layout(set = 0, binding = 2) buffer buf_out_a { uint out_a[]; };
|
||
|
layout(set = 0, binding = 3) buffer buf_out_b { uint out_b[]; };
|
||
|
|
||
|
// Kompute supports push constants updated on dispatch
|
||
|
layout(push_constant) uniform PushConstants {
|
||
|
float val;
|
||
|
} push_const;
|
||
|
|
||
|
// Kompute also supports spec constants on initalization
|
||
|
layout(constant_id = 0) const float const_one = 0;
|
||
|
|
||
|
void main() {
|
||
|
uint index = gl_GlobalInvocationID.x;
|
||
|
out_a[index] += uint( in_a[index] * in_b[index] );
|
||
|
out_b[index] += uint( const_one * push_const.val );
|
||
|
}
|
||
|
)");
|
||
|
|
||
|
// Run the function declared above with our raw string shader
|
||
|
kompute(shader);
|
||
|
}
|
||
|
|
||
|
```
|
||
|
|
||
|
### Your First Kompute (Python)
|
||
|
|
||
|
The [Python package](https://kompute.cc/overview/python-package.html) provides a [high level interactive interface](https://kompute.cc/overview/python-reference.html) that enables for experimentation whilst ensuring high performance and fast development workflows.
|
||
|
|
||
|
```python
|
||
|
|
||
|
from .utils import compile_source # using util function from python/test/utils
|
||
|
|
||
|
def kompute(shader):
|
||
|
# 1. Create Kompute Manager with default settings (device 0, first queue and no extensions)
|
||
|
mgr = kp.Manager()
|
||
|
|
||
|
# 2. Create and initialise Kompute Tensors through manager
|
||
|
|
||
|
# Default tensor constructor simplifies creation of float values
|
||
|
tensor_in_a = mgr.tensor([2, 2, 2])
|
||
|
tensor_in_b = mgr.tensor([1, 2, 3])
|
||
|
# Explicit type constructor supports uint32, int32, double, float and bool
|
||
|
tensor_out_a = mgr.tensor_t(np.array([0, 0, 0], dtype=np.uint32))
|
||
|
tensor_out_b = mgr.tensor_t(np.array([0, 0, 0], dtype=np.uint32))
|
||
|
|
||
|
params = [tensor_in_a, tensor_in_b, tensor_out_a, tensor_out_b]
|
||
|
|
||
|
# 3. Create algorithm based on shader (supports buffers & push/spec constants)
|
||
|
workgroup = (3, 1, 1)
|
||
|
spec_consts = [2]
|
||
|
push_consts_a = [2]
|
||
|
push_consts_b = [3]
|
||
|
|
||
|
# See documentation shader section for compile_source
|
||
|
spirv = compile_source(shader)
|
||
|
|
||
|
algo = mgr.algorithm(params, spirv, workgroup, spec_consts, push_consts_a)
|
||
|
|
||
|
# 4. Run operation synchronously using sequence
|
||
|
(mgr.sequence()
|
||
|
.record(kp.OpTensorSyncDevice(params))
|
||
|
.record(kp.OpAlgoDispatch(algo)) # Binds default push consts provided
|
||
|
.eval() # evaluates the two recorded ops
|
||
|
.record(kp.OpAlgoDispatch(algo, push_consts_b)) # Overrides push consts
|
||
|
.eval()) # evaluates only the last recorded op
|
||
|
|
||
|
# 5. Sync results from the GPU asynchronously
|
||
|
sq = mgr.sequence()
|
||
|
sq.eval_async(kp.OpTensorSyncLocal(params))
|
||
|
|
||
|
# ... Do other work asynchronously whilst GPU finishes
|
||
|
|
||
|
sq.eval_await()
|
||
|
|
||
|
# Prints the first output which is: { 4, 8, 12 }
|
||
|
print(tensor_out_a)
|
||
|
# Prints the first output which is: { 10, 10, 10 }
|
||
|
print(tensor_out_b)
|
||
|
|
||
|
if __name__ == "__main__":
|
||
|
|
||
|
# Define a raw string shader (or use the Kompute tools to compile to SPIRV / C++ header
|
||
|
# files). This shader shows some of the main components including constants, buffers, etc
|
||
|
shader = """
|
||
|
#version 450
|
||
|
|
||
|
layout (local_size_x = 1) in;
|
||
|
|
||
|
// The input tensors bind index is relative to index in parameter passed
|
||
|
layout(set = 0, binding = 0) buffer buf_in_a { float in_a[]; };
|
||
|
layout(set = 0, binding = 1) buffer buf_in_b { float in_b[]; };
|
||
|
layout(set = 0, binding = 2) buffer buf_out_a { uint out_a[]; };
|
||
|
layout(set = 0, binding = 3) buffer buf_out_b { uint out_b[]; };
|
||
|
|
||
|
// Kompute supports push constants updated on dispatch
|
||
|
layout(push_constant) uniform PushConstants {
|
||
|
float val;
|
||
|
} push_const;
|
||
|
|
||
|
// Kompute also supports spec constants on initalization
|
||
|
layout(constant_id = 0) const float const_one = 0;
|
||
|
|
||
|
void main() {
|
||
|
uint index = gl_GlobalInvocationID.x;
|
||
|
out_a[index] += uint( in_a[index] * in_b[index] );
|
||
|
out_b[index] += uint( const_one * push_const.val );
|
||
|
}
|
||
|
"""
|
||
|
|
||
|
kompute(shader)
|
||
|
|
||
|
```
|
||
|
|
||
|
### Interactive Notebooks & Hands on Videos
|
||
|
|
||
|
You are able to try out the interactive Colab Notebooks which allow you to use a free GPU. The available examples are the Python and C++ examples below:
|
||
|
|
||
|
<table>
|
||
|
<tr>
|
||
|
|
||
|
<td width="50%">
|
||
|
<h5>Try the interactive <a href="https://colab.research.google.com/drive/1l3hNSq2AcJ5j2E3YIw__jKy5n6M615GP?usp=sharing">C++ Colab</a> from <a href="https://towardsdatascience.com/machine-learning-and-data-processing-in-the-gpu-with-vulkan-kompute-c9350e5e5d3a">Blog Post</a></h5>
|
||
|
</td>
|
||
|
|
||
|
<td>
|
||
|
<h5>Try the interactive <a href="https://colab.research.google.com/drive/15uQ7qMZuOyk8JcXF-3SB2R5yNFW21I4P">Python Colab</a> from <a href="https://towardsdatascience.com/beyond-cuda-gpu-accelerated-python-for-machine-learning-in-cross-vendor-graphics-cards-made-simple-6cc828a45cc3">Blog Post</a></h5>
|
||
|
</td>
|
||
|
|
||
|
</tr>
|
||
|
<tr>
|
||
|
|
||
|
<td width="50%">
|
||
|
<a href="https://colab.research.google.com/drive/1l3hNSq2AcJ5j2E3YIw__jKy5n6M615GP?authuser=1#scrollTo=1BipBsO-fQRD">
|
||
|
<img src="https://raw.githubusercontent.com/KomputeProject/kompute/master/docs/images/binder-cpp.jpg">
|
||
|
</a>
|
||
|
</td>
|
||
|
|
||
|
<td>
|
||
|
<a href="https://colab.research.google.com/drive/15uQ7qMZuOyk8JcXF-3SB2R5yNFW21I4P">
|
||
|
<img src="https://raw.githubusercontent.com/KomputeProject/kompute/master/docs/images/binder-python.jpg">
|
||
|
</a>
|
||
|
</td>
|
||
|
|
||
|
</tr>
|
||
|
</table>
|
||
|
|
||
|
|
||
|
You can also check out the two following talks presented at the FOSDEM 2021 conference.
|
||
|
|
||
|
Both videos have timestamps which will allow you to skip to the most relevant section for you - the intro & motivations for both is almost the same so you can skip to the more specific content.
|
||
|
|
||
|
<table>
|
||
|
<tr>
|
||
|
|
||
|
<td width="50%">
|
||
|
<h5>Watch the video for <a href="https://www.youtube.com/watch?v=Xz4fiQNmGSA">C++ Enthusiasts</a> </h5>
|
||
|
</td>
|
||
|
|
||
|
<td>
|
||
|
<h5>Watch the video for <a href="https://www.youtube.com/watch?v=AJRyZ09IUdg">Python & Machine Learning</a> Enthusiasts</h5>
|
||
|
</td>
|
||
|
|
||
|
</tr>
|
||
|
<tr>
|
||
|
|
||
|
<td width="50%">
|
||
|
<a href="https://www.youtube.com/watch?v=Xz4fiQNmGSA">
|
||
|
<img src="https://raw.githubusercontent.com/KomputeProject/kompute/master/docs/images/kompute-cpp-video.png">
|
||
|
</a>
|
||
|
</td>
|
||
|
|
||
|
<td>
|
||
|
<a href="https://www.youtube.com/watch?v=AJRyZ09IUdg">
|
||
|
<img src="https://raw.githubusercontent.com/KomputeProject/kompute/master/docs/images/kompute-python-video.png">
|
||
|
</a>
|
||
|
</td>
|
||
|
|
||
|
</tr>
|
||
|
</table>
|
||
|
|
||
|
|
||
|
## Architectural Overview
|
||
|
|
||
|
The core architecture of Kompute includes the following:
|
||
|
* [Kompute Manager](https://kompute.cc/overview/reference.html#manager) - Base orchestrator which creates and manages device and child components
|
||
|
* [Kompute Sequence](https://kompute.cc/overview/reference.html#sequence) - Container of operations that can be sent to GPU as batch
|
||
|
* [Kompute Operation (Base)](https://kompute.cc/overview/reference.html#algorithm) - Base class from which all operations inherit
|
||
|
* [Kompute Tensor](https://kompute.cc/overview/reference.html#tensor) - Tensor structured data used in GPU operations
|
||
|
* [Kompute Algorithm](https://kompute.cc/overview/reference.html#algorithm) - Abstraction for (shader) logic executed in the GPU
|
||
|
|
||
|
To see a full breakdown you can read further in the [C++ Class Reference](https://kompute.cc/overview/reference.html).
|
||
|
|
||
|
<table>
|
||
|
<th>
|
||
|
Full Architecture
|
||
|
</th>
|
||
|
<th>
|
||
|
Simplified Kompute Components
|
||
|
</th>
|
||
|
<tr>
|
||
|
<td width=30%>
|
||
|
|
||
|
|
||
|
<img width="100%" src="https://raw.githubusercontent.com/KomputeProject/kompute/master/docs/images/kompute-vulkan-architecture.jpg">
|
||
|
|
||
|
<br>
|
||
|
<br>
|
||
|
(very tiny, check the <a href="https://ethicalml.github.io/vulkan-kompute/overview/reference.html">full reference diagram in docs for details</a>)
|
||
|
<br>
|
||
|
<br>
|
||
|
|
||
|
<img width="100%" src="https://raw.githubusercontent.com/KomputeProject/kompute/master/docs/images/suspicious.jfif">
|
||
|
|
||
|
</td>
|
||
|
<td>
|
||
|
<img width="100%" src="https://raw.githubusercontent.com/KomputeProject/kompute/master/docs/images/kompute-architecture.jpg">
|
||
|
</td>
|
||
|
</tr>
|
||
|
</table>
|
||
|
|
||
|
|
||
|
## Asynchronous and Parallel Operations
|
||
|
|
||
|
Kompute provides flexibility to run operations in an asynrchonous way through vk::Fences. Furthermore, Kompute enables for explicit allocation of queues, which allow for parallel execution of operations across queue families.
|
||
|
|
||
|
The image below provides an intuition on how Kompute Sequences can be allocated to different queues to enable parallel execution based on hardware. You can see the [hands on example](https://kompute.cc/overview/advanced-examples.html#parallel-operations), as well as the [detailed documentation page](https://kompute.cc/overview/async-parallel.html) describing how it would work using an NVIDIA 1650 as an example.
|
||
|
|
||
|
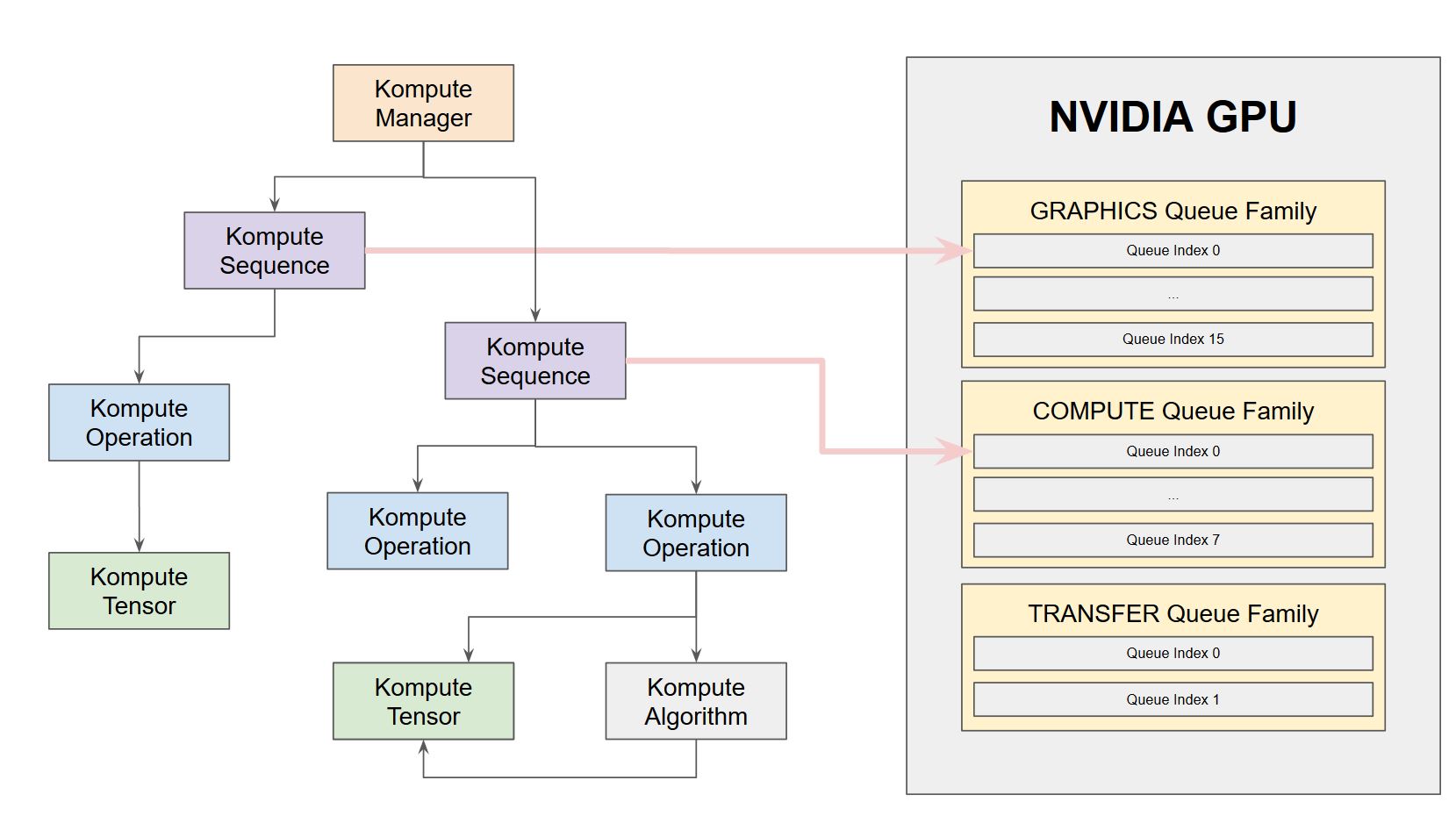
|
||
|
|
||
|
## Mobile Enabled
|
||
|
|
||
|
Kompute has been optimized to work in mobile environments. The [build system](#build-overview) enables for dynamic loading of the Vulkan shared library for Android environments, together with a working [Android NDK wrapper](https://github.com/KomputeProject/kompute/tree/master/vk_ndk_wrapper_include) for the CPP headers.
|
||
|
|
||
|
<table>
|
||
|
<tr>
|
||
|
|
||
|
<td width="70%">
|
||
|
<p>
|
||
|
For a full deep dive you can read the blog post "<a href="https://towardsdatascience.com/gpu-accelerated-machine-learning-in-your-mobile-applications-using-the-android-ndk-vulkan-kompute-1e9da37b7617">Supercharging your Mobile Apps with On-Device GPU Accelerated Machine Learning</a>".
|
||
|
|
||
|
You can also access the <a href="https://github.com/KomputeProject/kompute/tree/v0.4.0/examples/android/android-simple">end-to-end example code</a> in the repository, which can be run using android studio.
|
||
|
|
||
|
</p>
|
||
|
|
||
|
|
||
|
<img src="https://raw.githubusercontent.com/KomputeProject/kompute/android-example/docs/images/android-editor.jpg">
|
||
|
|
||
|
</td>
|
||
|
|
||
|
|
||
|
<td width="30%">
|
||
|
<img src="https://raw.githubusercontent.com/KomputeProject/kompute/android-example/docs/images/android-kompute.jpg">
|
||
|
</td>
|
||
|
|
||
|
</tr>
|
||
|
</table>
|
||
|
|
||
|
## More examples
|
||
|
|
||
|
### Simple examples
|
||
|
|
||
|
* [Simple multiplication example](https://kompute.cc/overview/advanced-examples.html#simple-shader-example)
|
||
|
* [Record batch commands with a Kompute Sequence](https://kompute.cc/overview/advanced-examples.html#record-batch-commands)
|
||
|
* [Run Asynchronous Operations](https://kompute.cc/overview/advanced-examples.html#asynchronous-operations)
|
||
|
* [Run Parallel Operations Across Multiple GPU Queues](https://kompute.cc/overview/advanced-examples.html#parallel-operations)
|
||
|
* [Create your custom Kompute Operations](https://kompute.cc/overview/advanced-examples.html#your-custom-kompute-operation)
|
||
|
* [Implementing logistic regression from scratch](https://kompute.cc/overview/advanced-examples.html#logistic-regression-example)
|
||
|
|
||
|
### End-to-end examples
|
||
|
|
||
|
* [Machine Learning Logistic Regression Implementation](https://towardsdatascience.com/machine-learning-and-data-processing-in-the-gpu-with-vulkan-kompute-c9350e5e5d3a)
|
||
|
* [Parallelizing GPU-intensive Workloads via Multi-Queue Operations](https://towardsdatascience.com/parallelizing-heavy-gpu-workloads-via-multi-queue-operations-50a38b15a1dc)
|
||
|
* [Android NDK Mobile Kompute ML Application](https://towardsdatascience.com/gpu-accelerated-machine-learning-in-your-mobile-applications-using-the-android-ndk-vulkan-kompute-1e9da37b7617)
|
||
|
* [Game Development Kompute ML in Godot Engine](https://towardsdatascience.com/supercharging-game-development-with-gpu-accelerated-ml-using-vulkan-kompute-the-godot-game-engine-4e75a84ea9f0)
|
||
|
|
||
|
## Python Package
|
||
|
|
||
|
Besides the C++ core SDK you can also use the Python package of Kompute, which exposes the same core functionality, and supports interoperability with Python objects like Lists, Numpy Arrays, etc.
|
||
|
|
||
|
The only dependencies are Python 3.5+ and Cmake 3.4.1+. You can install Kompute from the [Python pypi package](https://pypi.org/project/kp/) using the following command.
|
||
|
|
||
|
```
|
||
|
pip install kp
|
||
|
```
|
||
|
|
||
|
You can also install from master branch using:
|
||
|
|
||
|
```
|
||
|
pip install git+git://github.com/KomputeProject/kompute.git@master
|
||
|
```
|
||
|
|
||
|
For further details you can read the [Python Package documentation](https://kompute.cc/overview/python-package.html) or the [Python Class Reference documentation](https://kompute.cc/overview/python-reference.html).
|
||
|
|
||
|
## C++ Build Overview
|
||
|
|
||
|
The build system provided uses `cmake`, which allows for cross platform builds.
|
||
|
|
||
|
The top level `Makefile` provides a set of optimized configurations for development as well as the docker image build, but you can start a build with the following command:
|
||
|
|
||
|
```
|
||
|
cmake -Bbuild
|
||
|
```
|
||
|
|
||
|
You also are able to add Kompute in your repo with `add_subdirectory` - the [Android example CMakeLists.txt file](https://github.com/KomputeProject/kompute/blob/7c8c0eeba2cdc098349fcd999102bb2cca1bf711/examples/android/android-simple/app/src/main/cpp/CMakeLists.txt#L3) shows how this would be done.
|
||
|
|
||
|
For a more advanced overview of the build configuration check out the [Build System Deep Dive](https://kompute.cc/overview/build-system.html) documentation.
|
||
|
|
||
|
## Kompute Development
|
||
|
|
||
|
We appreciate PRs and Issues. If you want to contribute try checking the "Good first issue" tag, but even using Kompute and reporting issues is a great contribution!
|
||
|
|
||
|
### Contributing
|
||
|
|
||
|
#### Dev Dependencies
|
||
|
|
||
|
* Testing
|
||
|
+ GTest
|
||
|
* Documentation
|
||
|
+ Doxygen (with Dot)
|
||
|
+ Sphynx
|
||
|
|
||
|
#### Development
|
||
|
|
||
|
* Follows Mozilla C++ Style Guide https://www-archive.mozilla.org/hacking/mozilla-style-guide.html
|
||
|
+ Uses post-commit hook to run the linter, you can set it up so it runs the linter before commit
|
||
|
+ All dependencies are defined in vcpkg.json
|
||
|
* Uses cmake as build system, and provides a top level makefile with recommended command
|
||
|
* Uses xxd (or xxd.exe windows 64bit port) to convert shader spirv to header files
|
||
|
* Uses doxygen and sphinx for documentation and autodocs
|
||
|
* Uses vcpkg for finding the dependencies, it's the recommended set up to retrieve the libraries
|
||
|
|
||
|
If you want to run with debug layers you can add them with the `KOMPUTE_ENV_DEBUG_LAYERS` parameter as:
|
||
|
|
||
|
```
|
||
|
export KOMPUTE_ENV_DEBUG_LAYERS="VK_LAYER_LUNARG_api_dump"
|
||
|
```
|
||
|
|
||
|
##### Updating documentation
|
||
|
|
||
|
To update the documentation you will need to:
|
||
|
* Run the gendoxygen target in the build system
|
||
|
* Run the gensphynx target in the build-system
|
||
|
* Push to github pages with `make push_docs_to_ghpages`
|
||
|
|
||
|
##### Running tests
|
||
|
|
||
|
Running the unit tests has been significantly simplified for contributors.
|
||
|
|
||
|
The tests run on CPU, and can be triggered using the ACT command line interface (https://github.com/nektos/act) - once you install the command line (And start the Docker daemon) you just have to type:
|
||
|
|
||
|
```
|
||
|
$ act
|
||
|
|
||
|
[Python Tests/python-tests] 🚀 Start image=axsauze/kompute-builder:0.2
|
||
|
[C++ Tests/cpp-tests ] 🚀 Start image=axsauze/kompute-builder:0.2
|
||
|
[C++ Tests/cpp-tests ] 🐳 docker run image=axsauze/kompute-builder:0.2 entrypoint=["/usr/bin/tail" "-f" "/dev/null"] cmd=[]
|
||
|
[Python Tests/python-tests] 🐳 docker run image=axsauze/kompute-builder:0.2 entrypoint=["/usr/bin/tail" "-f" "/dev/null"] cmd=[]
|
||
|
...
|
||
|
```
|
||
|
|
||
|
The repository contains unit tests for the C++ and Python code, and can be found under the `test/` and `python/test` folder.
|
||
|
|
||
|
The tests are currently run through the CI using Github Actions. It uses the images found in `docker-builders/`.
|
||
|
|
||
|
In order to minimise hardware requirements the tests can run without a GPU, directly in the CPU using [Swiftshader](https://github.com/google/swiftshader).
|
||
|
|
||
|
For more information on how the CI and tests are setup, you can go to the [CI, Docker and Tests Section](https://kompute.cc/overview/ci-tests.html) in the documentation.
|
||
|
|
||
|
## Motivations
|
||
|
|
||
|
This project started after seeing that a lot of new and renowned ML & DL projects like Pytorch, Tensorflow, Alibaba DNN, Tencent NCNN - among others - have either integrated or are looking to integrate the Vulkan SDK to add mobile (and cross-vendor) GPU support.
|
||
|
|
||
|
The Vulkan SDK offers a great low level interface that enables for highly specialized optimizations - however it comes at a cost of highly verbose code which requires 500-2000 lines of code to even begin writing application code. This has resulted in each of these projects having to implement the same baseline to abstract the non-compute related features of the Vulkan SDK. This large amount of non-standardised boiler-plate can result in limited knowledge transfer, higher chance of unique framework implementation bugs being introduced, etc.
|
||
|
|
||
|
We are currently developing Kompute not to hide the Vulkan SDK interface (as it's incredibly well designed) but to augment it with a direct focus on the Vulkan SDK's GPU computing capabilities. [This article](https://towardsdatascience.com/machine-learning-and-data-processing-in-the-gpu-with-vulkan-kompute-c9350e5e5d3a) provides a high level overview of the motivations of Kompute, together with a set of hands on examples that introduce both GPU computing as well as the core Kompute architecture.
|